În cadrul cursului Microsoft DP-100 – Designing and Implementing a Data Science Solution on Azure de 4 zile, participanții vor învăța cum să lucreze cu soluțiile de machine learning la scala cloud utilizând Azure Machine Learning.
Acest curs îi învață pe studenți cum să folosească noțiunile despre Python pe care le dețin deja pentru a gestiona datele, pentru a dezvolta si implementa modelul și monitoriza soluțiile de învățare automată în Microsoft Azure.
Acest curs se adresează pentru data scientists care dețin cunoștințe despre Python și framework-urile machine learning precum Scikit-Learn, PyTorch și Tensorflow care își doresc să construiască soluții de învățare automată în cloud.
Înainte de a participa la acest curs, studentul trebuie:
- aibă cunoștințe fundamentale de Microsoft Azure.
- aibă experiență în scrierea codului Python pentru a lucra cu datele folosind biblioteci precum Numpy, Pandas și Matplotlib.
- înțeleagă știința datelor; inclusiv modul de pregătire a datelor și instruirea modelelor de învățare a mașinilor folosind biblioteci de învățare automată cum ar fi Scikit-Learn, PyTorch sau Tensorflow.
Module 1: Introduction to Azure Machine Learning
In this module, you will learn how to provision an Azure Machine Learning workspace and use it to manage machine learning assets such as data, compute, model training code, logged metrics, and trained models. You will learn how to use the web-based Azure Machine Learning studio interface as well as the Azure Machine Learning SDK and developer tools like Visual Studio Code and Jupyter Notebooks to work with the assets in your workspace.
Lessons
- Getting Started with Azure Machine Learning
- Azure Machine Learning Tools
Lab : Creating an Azure Machine Learning Workspace
Lab : Working with Azure Machine Learning Tools
After completing this module, you will be able to
- Provision an Azure Machine Learning workspace
- Use tools and code to work with Azure Machine Learning
Module 2: No-Code Machine Learning with Designer
This module introduces the Designer tool, a drag and drop interface for creating machine learning models without writing any code. You will learn how to create a training pipeline that encapsulates data preparation and model training, and then convert that training pipeline to an inference pipeline that can be used to predict values from new data, before finally deploying the inference pipeline as a service for client applications to consume.
Lessons
- Training Models with Designer
- Publishing Models with Designer
Lab : Creating a Training Pipeline with the Azure ML Designer
Lab : Deploying a Service with the Azure ML Designer
After completing this module, you will be able to
- Use designer to train a machine learning model
- Deploy a Designer pipeline as a service
Module 3: Running Experiments and Training Models
In this module, you will get started with experiments that encapsulate data processing and model training code, and use them to train machine learning models.
Lessons
- Introduction to Experiments
- Training and Registering Models
Lab : Running Experiments
Lab : Training and Registering Models
After completing this module, you will be able to
- Run code-based experiments in an Azure Machine Learning workspace
- Train and register machine learning models
Module 4: Working with Data
Data is a fundamental element in any machine learning workload, so in this module, you will learn how to create and manage datastores and datasets in an Azure Machine Learning workspace, and how to use them in model training experiments.
Lessons
- Working with Datastores
- Working with Datasets
Lab : Working with Datastores
Lab : Working with Datasets
After completing this module, you will be able to
- Create and consume datastores
- Create and consume datasets
Module 5: Compute Contexts
One of the key benefits of the cloud is the ability to leverage compute resources on demand, and use them to scale machine learning processes to an extent that would be infeasible on your own hardware. In this module, you’ll learn how to manage experiment environments that ensure consistent runtime consistency for experiments, and how to create and use compute targets for experiment runs.
Lessons
- Working with Environments
- Working with Compute Targets
Lab : Working with Environments
Lab : Working with Compute Targets
After completing this module, you will be able to
- Create and use environments
- Create and use compute targets
Module 6: Orchestrating Operations with Pipelines
Now that you understand the basics of running workloads as experiments that leverage data assets and compute resources, it’s time to learn how to orchestrate these workloads as pipelines of connected steps. Pipelines are key to implementing an effective Machine Learning Operationalization (ML Ops) solution in Azure, so you’ll explore how to define and run them in this module.
Lessons
- Introduction to Pipelines
- Publishing and Running Pipelines
Lab : Creating a Pipeline
Lab : Publishing a Pipeline
After completing this module, you will be able to
- Create pipelines to automate machine learning workflows
- Publish and run pipeline services
Module 7: Deploying and Consuming Models
Models are designed to help decision making through predictions, so they’re only useful when deployed and available for an application to consume. In this module learn how to deploy models for real-time inferencing, and for batch inferencing.
Lessons
- Real-time Inferencing
- Batch Inferencing
Lab : Creating a Real-time Inferencing Service
Lab : Creating a Batch Inferencing Service
After completing this module, you will be able to
- Publish a model as a real-time inference service
- Publish a model as a batch inference service
Module 8: Training Optimal Models
By this stage of the course, you’ve learned the end-to-end process for training, deploying, and consuming machine learning models; but how do you ensure your model produces the best predictive outputs for your data? In this module, you’ll explore how you can use hyperparameter tuning and automated machine learning to take advantage of cloud-scale compute and find the best model for your data.
Lessons
- Hyperparameter Tuning
- Automated Machine Learning
Lab : Tuning Hyperparameters
Lab : Using Automated Machine Learning
After completing this module, you will be able to
- Optimize hyperparameters for model training
- Use automated machine learning to find the optimal model for your data
Module 9: Interpreting Models
Many of the decisions made by organizations and automated systems today are based on predictions made by machine learning models. It’s increasingly important to be able to understand the factors that influence the predictions made by a model, and to be able to determine any unintended biases in the model’s behavior. This module describes how you can interpret models to explain how feature importance determines their predictions.
Lessons
- Introduction to Model Interpretation
- using Model Explainers
Lab : Reviewing Automated Machine Learning Explanations
Lab : Interpreting Models
After completing this module, you will be able to
- Generate model explanations with automated machine learning
- Use explainers to interpret machine learning models
Module 10: Monitoring Models
After a model has been deployed, it’s important to understand how the model is being used in production, and to detect any degradation in its effectiveness due to data drift. This module describes techniques for monitoring models and their data.
Lessons
- Monitoring Models with Application Insights
- Monitoring Data Drift
Lab : Monitoring a Model with Application Insights
Lab : Monitoring Data Drift
After completing this module, you will be able to
- Use Application Insights to monitor a published model
- Monitor data drift
Acest curs îi pregătește pe cursanți pentru susținerea examenului Microsoft DP-100.
Microsoft DP-100 – Designing and Implementing a Data Science Solution on Azure
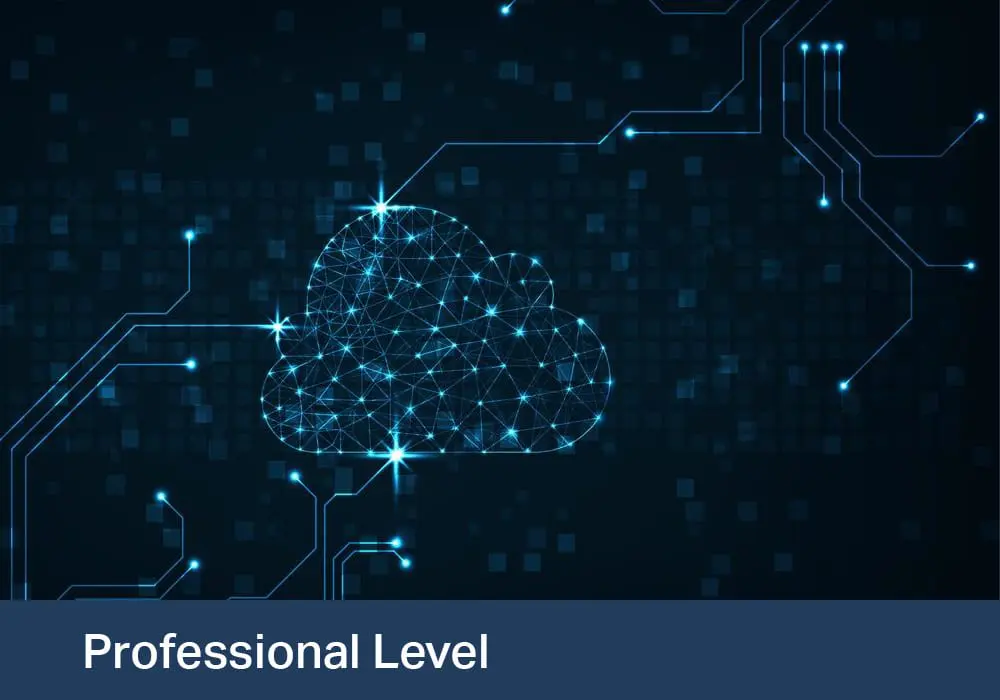
Detalii curs
FAQ curs Microsoft DP-100
Cum poate certificarea Microsoft DP-100 ajuta o companie să implementeze soluții eficiente de data science în Azure?
Certificarea DP-100 pregătește angajații să dezvolte și să implementeze soluții complete de data science utilizând Azure Machine Learning. Aceasta permite construirea de modele predictive eficiente, gestionarea datelor și aplicarea de algoritmi avansați, optimizând astfel procesele decizionale și îmbunătățind capacitatea de analiză a companiei.
Ce competențe vor dobândi angajații prin certificarea DP-100 care vor ajuta la optimizarea analizei datelor în companie?
Angajații vor învăța să gestioneze și să pregătească date la scară, să creeze și să antreneze modele de machine learning, și să implementeze soluții de data science folosind Azure. Aceste competențe permit analiza avansată a datelor și crearea de modele predictive care pot optimiza procesele de afaceri și îmbunătăți luarea deciziilor bazate pe date.
Cum poate certificarea DP-100 să ajute la îmbunătățirea procesului de luare a deciziilor în companie?
DP-100 ajută la dezvoltarea și implementarea modelelor predictive bazate pe date care pot oferi informații valoroase pentru deciziile strategice. Aceasta permite companiei să utilizeze machine learning pentru a anticipa tendințele, optimiza procesele și îmbunătăți rezultatele de afaceri prin decizii bazate pe analize precise.
Care sunt principalele tehnologii și servicii Azure acoperite în certificarea DP-100 și cum sunt acestea relevante pentru companie?
Certificarea DP-100 acoperă tehnologii și servicii esențiale precum Azure Machine Learning, Azure Databricks, Azure Synapse Analytics și gestionarea datelor. Aceste servicii permit colectarea, procesarea și analiza datelor la scară mare, oferind suport pentru modele predictive și soluții de machine learning care îmbunătățesc performanța și eficiența afacerii noastre.
De ce îmi este afișată această pagină?
Acest curs este recomandat datorită căutărilor tale ce includ termeni precum: dp100, mslearn dp100, microsoft azure data scientist associate dp 100, azure data scientist associate dp 100, dp 100 learning path, azure data scientist associate dp100, microsoft dp100, azure data scientist dp 100, dp 100 course, azure dp 100, dp 100 microsoft, azure dp 100 course, dp100 certification, dp 100 azure, microsoft azure data scientist associate dp 100 professional certificate, dp 100 azure data scientist, microsoft learn dp 100, dp 100 azure data scientist associate, azure certification dp 100, dp 100 certificate sau alții.